Latent Intrinsics Emerge from Training to Relight
Xiao Zhang, Will Gao, Seemandhar Jain, Michael Maire, David A. Forsyth, Anand Bhattad
Paper | Code (coming soon)
We learn an image relighting model representing image intrinsic property as latent variables, which has the following highlighted capabilities:
(1) Our relighting model is trained on paired relighted images, yet it generalizes to relighting images using any arbitrary reference.

(2) Without any supervision, our model could infer image albedo for free.

Method: Intrinsic representation via structural modelling
We implement our model as an autoencoder that internally separates extrinsic representation L and intrinsic representations S. We train the model to reconstruct the relighting target images using L and S inferred from relighted pairs. The extrinsic L is incorporated through constrained scaling, a structural regularization technique that explicitly regulates the information carried from L. This design enables us to learn a generalizable intrinsic representation, allowing us to relight images with arbitrary references and infer the albedo at no additional cost.
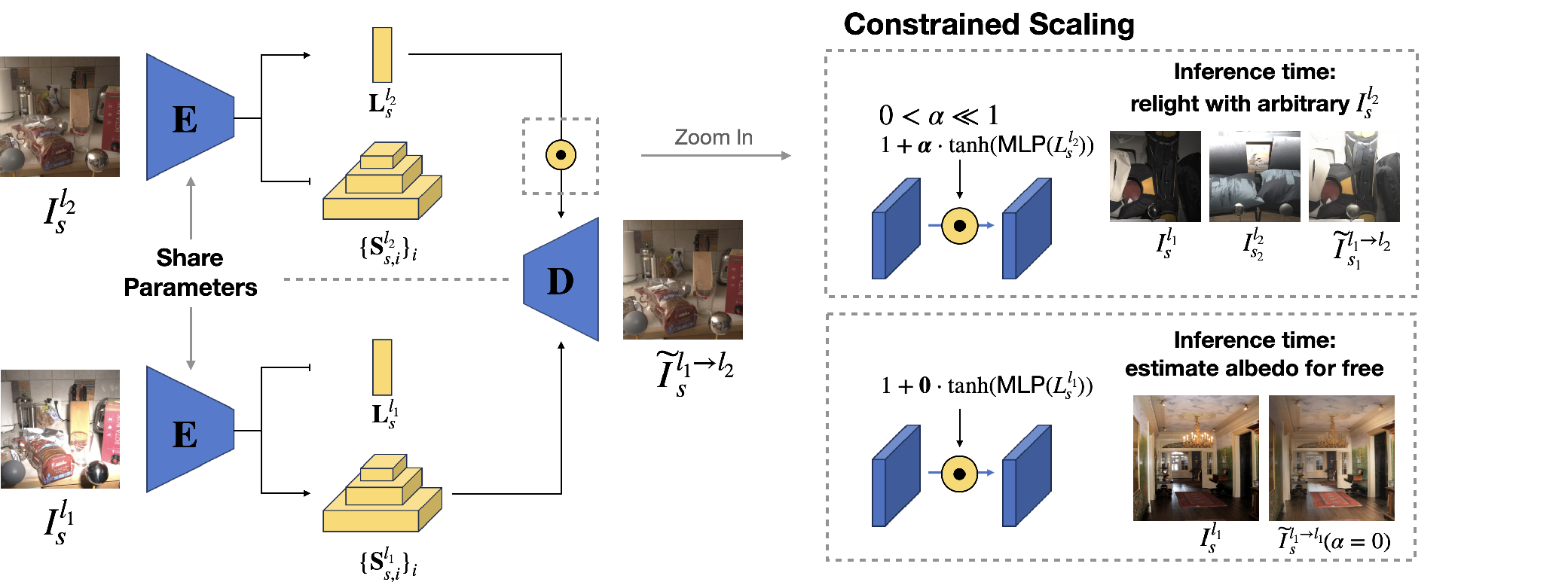
Results: Image Relighting with Arbitrary References
Without any supervision, our model accurately infers the light from the reference images. From a zoomed-in view of the chrome ball, our method effectively retains the intricate room layout and accurately renders the appropriate lighting patterns.
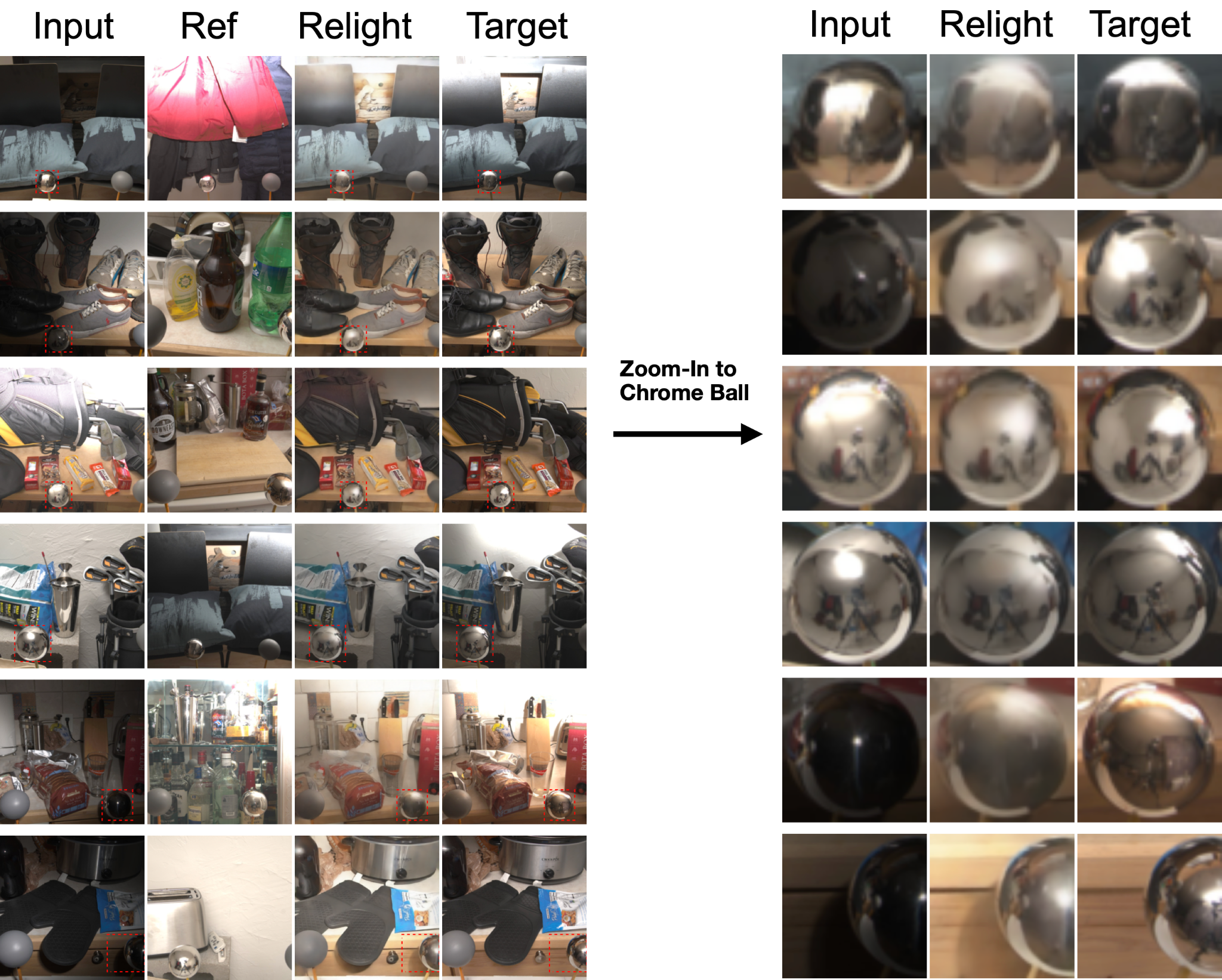
We train our model on relighted image pairs generated by StyLitGAN[1]. We demonstrate that our model learns to infer the semantic concept of adjusting the bedside light as a latent extrinsic representation, which can be used to relight the generated images.
In the bottom left examples, our model resists StyLitGAN's tendency to create unrealistic illuminations.
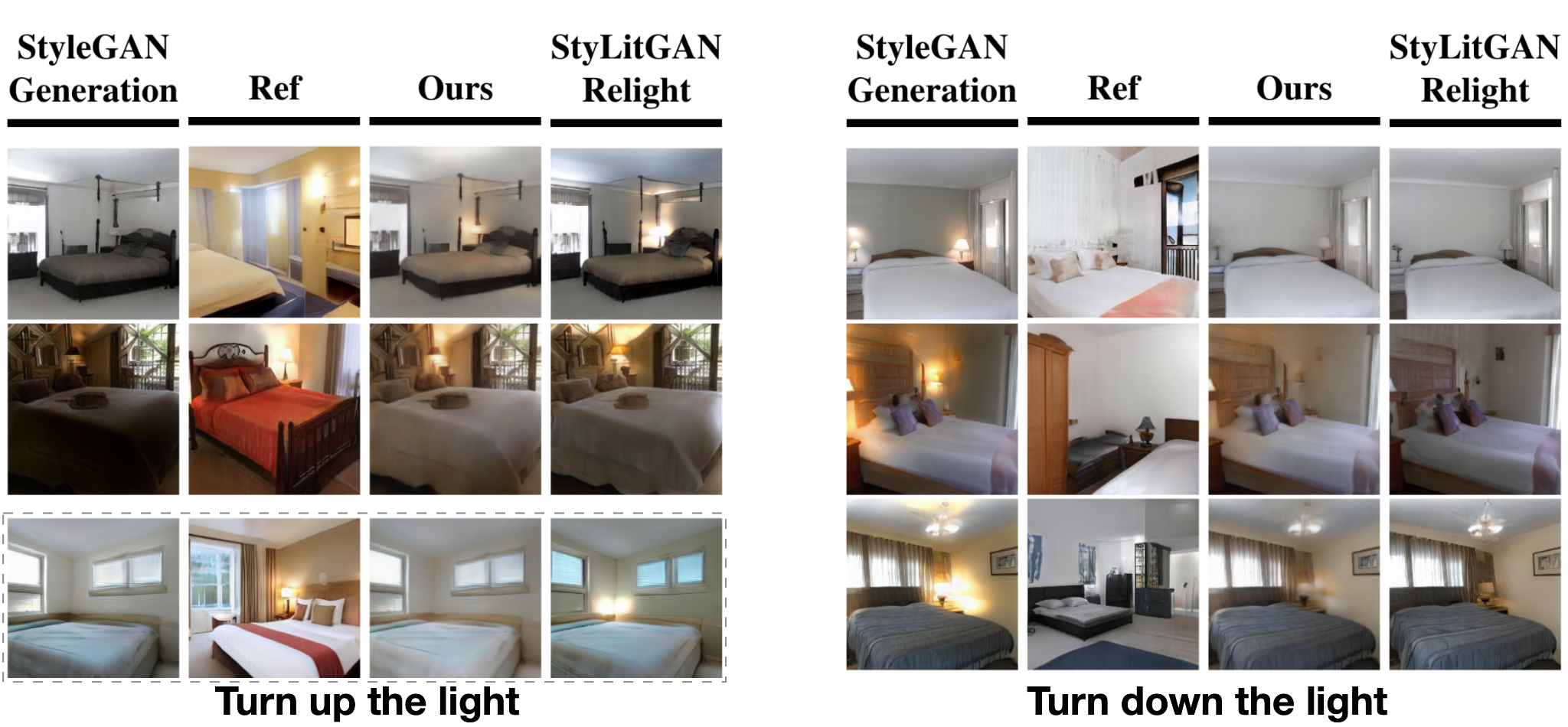
Results: Image Albedo Estimation
Without being trained with any form of albedo annotations, our method can infer albedo for complex scenes at no additional cost and achieves better performance compared to state-of-the-art supervised methods, Intrinsic Diffusion[2], which is trained on the CG dataset.
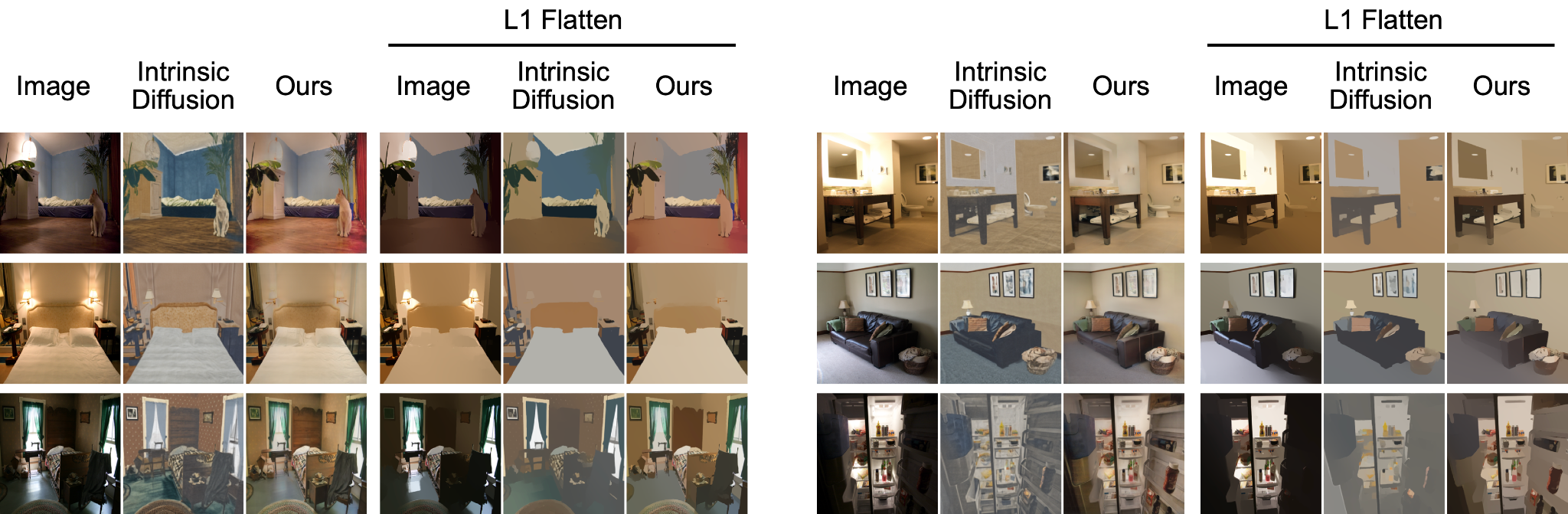
References:
[1] A. Bhattad, James Soole, D.A. Forsyth. StyLitGAN: Image-based Relighting via Latent Control.
[2] P. Kocsis, V. Sitzmann, M. Nießner. Intrinsic Image Diffusion for Indoor Single-view Material Estimation.
[3] S. Bi, X. Han, Y. Yu. An L1 image transform for edge-preserving smoothing and scene-level intrinsic decomposition.